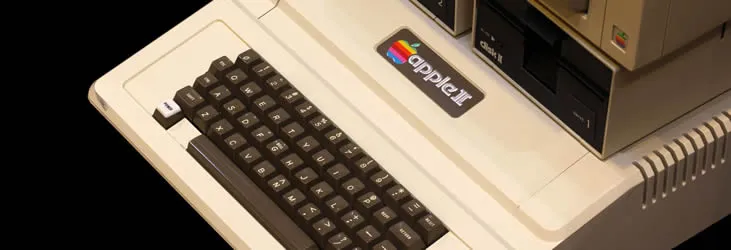
For decades, ever since Apple came out of the garage and made it fiscally feasible to automate office and factory tasks, we have heard computerized automation is coming to every corner of the workplace. To a degree, this automation has occurred, but not quite at the level that made front page news years ago. Now we hear that AI fueled robots and algorithms will take over the workplace of tomorrow, and many projects are rapidly trying to fulfill the promise that AI holds for the future.
While the pundits will scream about job loss in the short term, I’m far more concerned with a different problem. How well, how efficiently, will those automation processes be implemented? If what I have seen in the past is any indication, many of these automation rollouts will be incredibly expensive, and not accomplish their goal of work and time-saving computerized automation - at least in the first, second or even third iteration of the process.
I have watched my computer programming brethren for many years make the same mistakes over and over and over again. The normal project flow consists of the techs discussing the project and mapping it out with the management team, often never receiving input from the middle managers, and rarely giving more than a passing glance to the people actually doing that task.
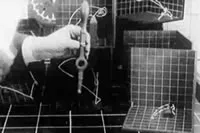
This was his starting point, the jumping off spot for any assembly line automation or clerical time saving process he would later present to management.
I spent many years working with clients who ran companies large and small, running the gamut from assembly lines, to transportation, to farming, and every office and education type imaginable. I would sit in meetings with management, listen carefully to what they thought they wanted to accomplish by computerizing their business, and then speak to the people who actually DID that job. I would sit behind them, asking a thousand questions, and at times even performing the job myself. Once I had a clear understanding of the tasks they performed and the bottlenecks they worked through on a daily basis, then and only then would I gather my team and sit down to plan the hardware and software needed to achieve true time saving and money saving upgrades to their systems.
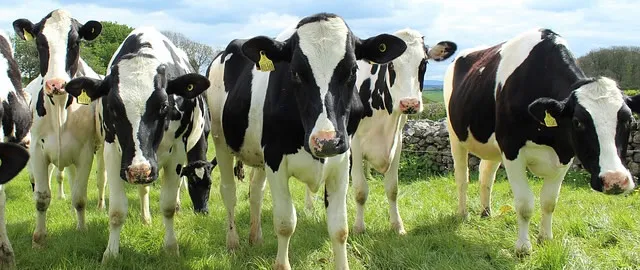
This has led me to “holding” many diverse jobs in my lifetime. I’ve milked cows, to study the milkhouse traffic flow, run a cash register at a convenient store to improve customer service and checkout counter efficiency, ridden hundreds of miles in 18 wheelers to study truck loading and delivery systems. I’ve “worked” in banks, copywriting pools in ad agencies, retail establishments, restaurants, and more. If I were to include these “jobs” on my resume they would number 47. Yes, I kept careful track of them. I am a stats junky and data nerd after all.
Without understanding what the job IS, thoroughly knowing each step of the process, and how it fits in to make the whole, any automating of that process is doomed to failure, at least in its first iteration. In all 47 of those jobs, I discovered that while the management might have come up “through the ranks”, the people actually performing the job had their own ways of dealing with the work flow that management rarely knew or understood. In many cases, management had no true idea of what the job actually entailed, or the steps that needed to be taken for successful completion of the task.
But AI faces problems that go beyond just not knowing what a job truly entails. There are also mechanical issues
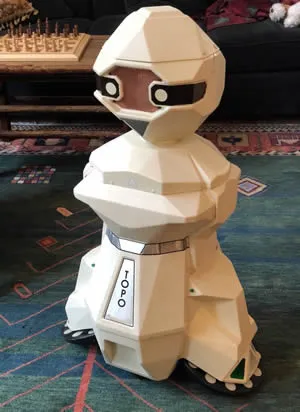
Then I waited expectantly for him to return to the living room with the tray, stopping in front of each guest as he had been programmed to do. Unfortunately his temperature sensors were something I had not taken into account and the little white machine turned and attempted to serve drinks to my oven which was finishing off cooking our meal and apparently giving off the same heat signal as the humans in the other room. I was anticipating “ooohs and ahhs” from my enthralled guests and instead got a hearty round of laughter and giggles.
Fast forward to today and AI robots are being implemented in futuristic growing buildings. If they are successful, and I have no doubt that they will be eventually, these buildings will go a long way to solving our global food problems. Bathed in the optimal amount of light, watered in exact amounts determined by sensor readouts, the grow time is decreased and the crop yield is maximized.
However, the two brothers behind this project ran into a problem that ncreased their development time and cost by at least two years. Sensors and computerized delivery systems for nutrients, sun and water were relatively easy to implement. But the weeding and harvesting by AI robots proved to be the sticking point.
The robot could easily lift hundreds of pounds, a necessary function when moving the trays full of plants to larger space that allowed the growing to continue. But the same robot had to be able to determine the difference between a plant and a weed, and be able to grasp tender shoots for transplanting without crushing them.
It also had to be able to harvest a variety of fruits and vegetables without bruising or harming the ripe plant.
Robotics.org details some of the obstacles involved with just harvesting one type of vegetable.
“a robotic system designed to pick sweet peppers encounters many obstacles. Vision systems have to determine the location and ripeness of the pepper in harsh conditions, including the presence of dust, varying light intensity, temperature swings and movement created by the wind."
"But it still takes more than advanced vision systems to pick a pepper. A robotic arm has to navigate environments with just as many obstacles to delicately grasp and place a pepper. This process is very different from picking and placing a metal part on an assembly line. The agricultural robotic arm must be flexible in a dynamic environment and accurate enough not to damage the peppers as they’re being picked.”
Combining all of these attributes into one AI mechanism is not simple. It will eventually be solved, just as office and factory automation have been solved in past decades. But regardless of the enormous strides being made in AI automation, there is still a long way to go to a fully roboticized office, factory floor, or farm. Studying and involving the people who actually do those jobs every day in the development process, could shave both development time and money from that process.